Original Data Sources
The DisGeNET database integrates information of human gene-disease associations (GDAs) and variant-disease associations (VDAs) from various repositories including Mendelian, complex and environmental diseases. The integration is performed by means of gene and disease vocabulary mapping and by using the DisGeNET association type ontology. For more details, see the original publications: Piñero et al., 2019; Piñero et al., 2016; Piñero et al., 2015; Bauer-Mehren et al., 2011 and Bauer-Mehren et al., 2010 .
Gene-Disease Associations
The gene-disease information in DisGeNET is organized according to the types of source databases:
- CURATED: GDAs from UniProt, PsyGeNET, Orphanet, the CGI, CTD (human data), ClinGen, and the Genomics England PanelApp.
- ANIMAL MODELS: GDAs from RGD, MGD, and CTD (mouse and rat data)
- INFERRED: GDAs from the Human Phenotype Ontology, and GDAs inferred from VDAs reported by Clinvar, the GWAS catalog and GWAS db
- ALL: GDAs from previous sources and from LHGDN and BeFree
Curated Data
These data contain GDAs provided by the expert curated resources.UNIPROT: UniProt/SwissProt is a database containing curated information about protein sequence, structure and function (The UniProt Consortium, 2018). GDAs were obtained from the humsavar file. We include only the associations marked as Disease.
CTD: The Comparative Toxicogenomics DatabaseTM contains manually curated information about gene-disease relationships with focus on understanding the effects of environmental chemicals on human health (Davis et al., 2018 ). From CTD we only include associations marked as marker/mechanism or therapeutic.
ORPHANET: Orphanet is the reference portal for information on rare diseases and orphan drugs, for all audiences (© INSERM 1997). Its aim is to help improve the diagnosis, care and treatment of patients with rare diseases. From Orphanet we keep all GDAs. CLINGEN: The Clinical Genome Resource is dedicated to building an authoritative central resource that defines the clinical relevance of genes and variants for use in precision medicine and research ( Rehm et al., 2018 ). From ClinGen, we do not include GDAs labeled as "refuted". GENOMICS ENGLAND: The Genomics England PanelApp is a publically available knowledge base that allows virtual gene panels related to human disorders to be created, stored and queried. From this source we keep all GDAs. CGI: The Cancer Genome Interpreter is a tool that (i) identifies known oncogenic alterations; (ii) predicts potential drivers among those of unknown significance and (iii) identify alterations in the tumor known to affect the response to anti-cancer drugs ( Tamborero et al., 2018 ). It also distributes the catalog of Cancer Driver Genes, which is a selection of genes driving tumorigenesis in a certain tumor type(s) upon a certain alteration (mutation, copy number alteration and/or gene translocation). From this dataset, we only keep validated data and do not include computational predictions.PSYGENET: PsyGeNET (Psychiatric disorders Gene association NETwork) is a resource for the exploratory analysis of psychiatric diseases and their associated genes (Gutiérrez-Sacristán et al., 2009 ).
Animal Models Data
These data include GDAs provided by the resources containing information about animal models (currently rat and mouse) of disease. We have used orthology information to map the associations to the human genes.CTD Rat and CTD Mouse: CTDTM data containing Rattus norvegicus and Mus musculus gene-disease associations.
MGD: The Mouse Genome Database is the international community resource for integrated genetic, genomic and biological data about the laboratory mouse (Smith et al., 2018 ). From MGD, we keep only the associations marked as mouse, laboratory.
RGD: The Rat Genome Database is a collaborative effort between leading research institutions involved in rat genetic and genomic research (Laulederkind et al., 2018 ). We did not include the associations labeled as resistance, or no association, nor the ones annotated with the following evidence codes Inferred from electronic annotation, Inferred from sequence or structural similarity and Non-traceable author statement.
Inferred Data
These data refer to GDAs inferred from HPO and from VDAs. In the case of HPO, GDAs are inferred from phenotype-disease and gene-disease associations via triangulation. In the case of VDAs, a GDA is created for each combination of gene annotated to the variant, disease annotated to the variant, publication and association type. In order to infer GDAs from VDAs, we only consider variants that map to gene coding sequences. The variant is assigned to a gene or genes using dbSNP mappings, and the the consequence type of the variant in the gene using VEP. Only variants including the following consequence types are considered: coding sequence variant, frameshift variant, inframe deletion, incomplete terminal codon variant, inframe insertion, intron variant, missense variant, splice acceptor variant, splice donor variant, protein altering variant, splice region variant, start lost, stop gained, start retained variant, stop lost, stop retained variant, and synonymous variant.HPO: The Human Phenotype Ontology aims to provide a standardized vocabulary of phenotypic abnormalities encountered in human disease (Köhler et al., 2018 ). From the HPO, we import the genes_to_phenotype file, FREQUENT_FEATURES.
CLINVAR: ClinVar is a freely accessible, public archive of reports of the relationships among medically relevant variants and phenotypes, with supporting evidence (Landrum et al., 2018 ). From Clinvar, we only import variant-disease associations with labels: Pathogenic, Likely pathogenic, risk factor, and Affects.
GWAS CATALOG: The NHGRI-EBI GWAS Catalog is a quality controlled, manually curated, literature-derived collection of all published genome-wide association studies assaying at least 100,000 SNPs and all SNP-trait associations. (MacArthur et al., 2017 ). From the GWAS catalog, we keep VDAs with p-values < 1.0 x10-6.
GWAS DB: The GWASdb provides comprehensive data curation and knowledge integration for GWAS significant trait/disease associated SNPs (Li et al., 2016 ). From the GWASdb, we keep association with p-values < 1.0 x10-6.
Literature Data
LHGDN: The literature-derived human gene-disease network (LHGDN) is a text mining derived database with focus on extracting and classifying gene-disease associations with respect to several biomolecular conditions. It uses a machine learning based algorithm to extract semantic gene-disease relations from a textual source of interest. The semantic gene-disease relations were extracted with F-measures of 78 (see Bundschus et al., 2008 for further details). More specifically, the textual source utilized here originates from Entrez Gene's GeneRIF (Gene Reference Into Function) database (Mitchell,et al., 2003 ). LHGDN was created based on a GeneRIF version from March 31st, 2009, consisting of 414241 phrases. These phrases were further restricted to the organism Homo sapiens, which resulted in a total of 178004 phrases. We extracted all data from LHGDN and classified the original associations using the DisGeNET association type ontology.
BEFREE: We extracted gene-disease associations from MEDLINE abstracts published between January 1970 and December 2019 using the BeFree system. BeFree is composed of a Biomedical Named Entity Recognition (BioNER) module to detect diseases and genes (Bravo et al., 2014 ) and a relation extraction module based on morphosyntactic information (Bravo et al., 2015 ).
Negations of associations were detected using patterns and key words. This information is taken into account to compute the Evidence Index
BeFree GDAs are classified as ‘Biomarker’, ‘Genetic Variation’, ‘PostTranslational Modification’ or ‘Altered Expression’.
Variant-Disease Associations
The variant-disease information in DisGeNET is organized according to the types of source databases:
- CURATED: VDAs from UniProt, ClinVar, the GWAS Catalog, and GWAS db
- ALL: VDAs from previous sources and from BeFree
Curated Data
UNIPROT: UniProt/SwissProt is a database containing curated information about protein sequence, structure and function (The UniProt Consortium, 2018). VDAs were obtained from the humsavar file. We include only the associations marked as Disease.
CLINVAR: ClinVar is a freely accessible, public archive of reports of the relationships among medically relevant variants and phenotypes, with supporting evidence (Landrum et al., 2018 ). From Clinvar, we only import variant-disease associations with labels: Pathogenic, Likely pathogenic, risk factor, and Affects.
GWAS CATALOG: The NHGRI-EBI GWAS Catalog is a quality controlled, manually curated, literature-derived collection of all published genome-wide association studies assaying at least 100,000 SNPs and all SNP-trait associations. (MacArthur et al., 2017 ). From the GWAS catalog, we keep VDAs with p-values < 1.0 x10-6.
GWAS DB: The GWASdb provides comprehensive data curation and knowledge integration for GWAS significant trait/disease associated SNPs (Li et al., 2016 ). From the GWASdb, we keep association with p-values < 1.0 x10-6.
Literature Data
-
We applied SETH
(Thomas et
al., 2016)
, a tool for the recognition of variations (SNPs) from text and
their subsequent normalization to dbSNP build 137, to identify
variant mentions. Then, the RE module of BeFree was applied to
identify variant-disease associations from MEDLINE sentences.
Disease-Disease Associations
The disease-disease associations (DDA) in DisGeNET have been obtained by computing the number of shared genes, and shared variants between pairs of diseases, by source. The DDAs can be explored from the Search panel by searching by disease (one or multiple diseases).
For each disease pair, we computed a Jaccard Index (JI), to assess the fraction of shared genes (or variants) among the diseases according to
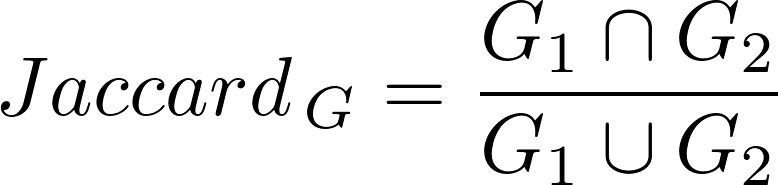
G1 is the set of genes associated to Disease 1
G2 is the set of genes associated to Disease 2
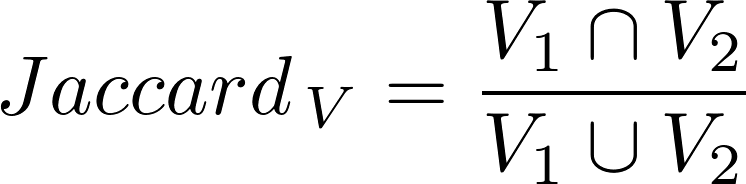
V1 is the set of variants associated to Disease 1
V2 is the set of variants associated to Disease 2
Database Statistics
The current version of DisGeNET (v7.0) contains 1135045 gene-disease associations (GDAs), between 21671 genes and 30170 diseases, disorders, traits, and clinical or abnormal human phenotypes, and 369554 variant-disease associations (VDAs), between 194515 variants and 14155 diseases, traits, and phenotypes. In the tables below, the distribution of the information provided by each source. The table also shows the number of evidences supporting the associations, that takes into account the number of publications and their association type.
Distribution of genes, diseases, and GDAs by source.
Source | Genes | Diseases | Assocs | Evidences |
CGI | 315 | 200 | 1557 | 1557 |
CLINGEN | 634 | 447 | 1260 | 7858 |
GENOMICS ENGLAND | 3967 | 6046 | 11215 | 18542 |
CTD_human | 8247 | 8246 | 67471 | 84380 |
ORPHANET | 3356 | 3266 | 6398 | 8322 |
PSYGENET | 1393 | 105 | 3296 | 6728 |
UNIPROT | 3894 | 3935 | 5728 | 17564 |
CURATED | 9703 | 11181 | 84038 | 137822 |
HPO | 4281 | 7591 | 164198 | 164198 |
CLINVAR | 4467 | 9247 | 26002 | 85646 |
GWASDB | 4862 | 450 | 11172 | 14663 |
GWASCAT | 10403 | 948 | 40443 | 56795 |
INFERRED | 13258 | 14843 | 233738 | 313885 |
CTD_mouse | 70 | 292 | 475 | 518 |
CTD_rat | 21 | 29 | 48 | 48 |
MGD | 1776 | 2085 | 4598 | 8569 |
RGD | 2143 | 1168 | 11667 | 13062 |
ANIMAL MODELS | 3334 | 3171 | 16660 | 22171 |
LHGDN | 5935 | 1793 | 31427 | 52794 |
BEFREE | 18839 | 17993 | 846474 | 2700332 |
LITERATURE | 18898 | 18171 | 858354 | 2738700 |
ALL | 21671 | 30170 | 1134942 | 3178358 |
Distribution of variants, diseases, and VDAs by source.
Source | Variants | Diseases | Assocs | Evidences |
CLINVAR | 72686 | 9354 | 117979 | 213927 |
GWASDB | 32660 | 479 | 47942 | 55715 |
GWASCAT | 61338 | 1026 | 89454 | 101157 |
UNIPROT | 22448 | 3697 | 23296 | 182304 |
CURATED | 168051 | 10413 | 261227 | 542672 |
BEFREE | 38206 | 6397 | 114628 | 186739 |
LITERATURE | 38206 | 6397 | 114628 | 186739 |
ALL | 194515 | 14155 | 369554 | 727366 |
* Diseases, phenotypes, and disease groups. See more information here
Distribution of clinical concepts, genes, and variant annotations according to the DisGeNET disease type.
Number of clinical concepts | Number of associated genes | Number of associated Variants | |
---|---|---|---|
disease | 21838 | 20163 | 139004 |
disease group | 962 | 15474 | 22477 |
phenotype | 7493 | 16854 | 62686 |
Distribution of clinical concepts, genes, and variant annotations according to the UMLS Semantic Type.

Distribution of clinical concepts, genes, and variant annotations according to the MSH Disease Class.

Distribution of protein classes.

Venn diagram representing the overlaps among the different types of global sources (curated, animal models, and text mining).

In Panel A, the GDAs, and in Panel B, the VDAs
DisGeNET Metrics
We have developed two scores to rank the gene-disease, and the variant-disease associations according to their level of evidence. These scores range from 0 to 1, and take into account the number and type of sources (level of curation, model organisms), and the number of publications supporting the association.
GDA Score
The DisGeNET Score (S) for GDAs is computed according to:
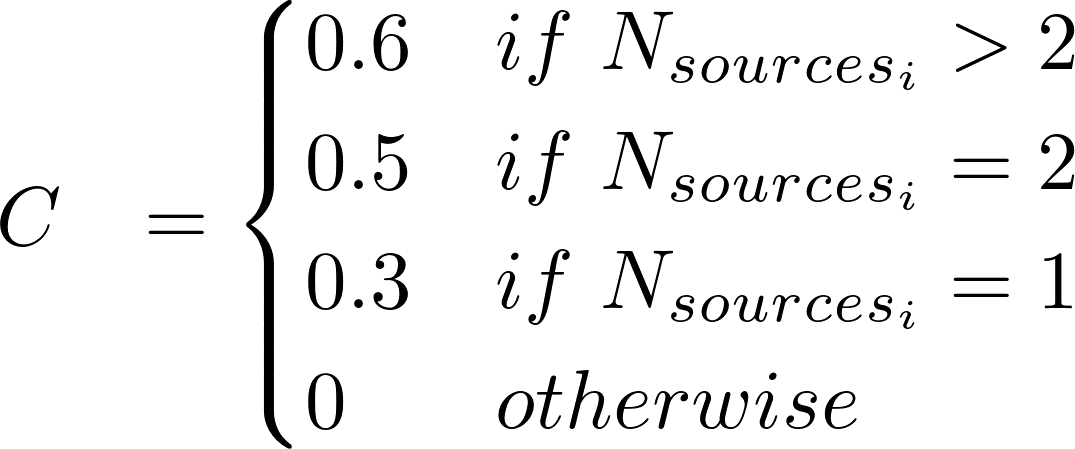
where:
Nsourcesi is the number of CURATED sources supporting a GDA
i ∈ CGI, CLINGEN, GENOMICS ENGLAND, CTD, PSYGENET, ORPHANET, UNIPROT
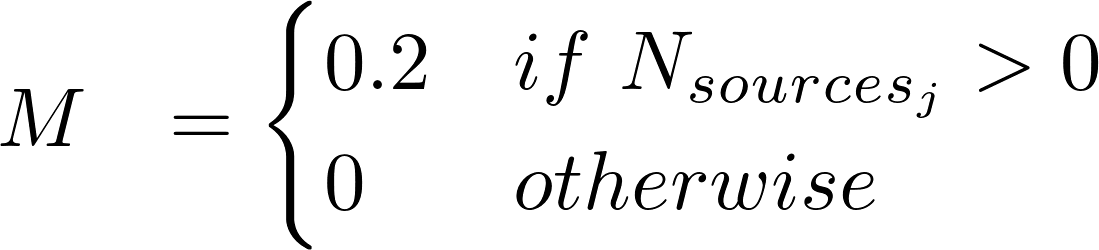
where:
j ∈ Rat, Mouse from RGD, MGD, and CTD
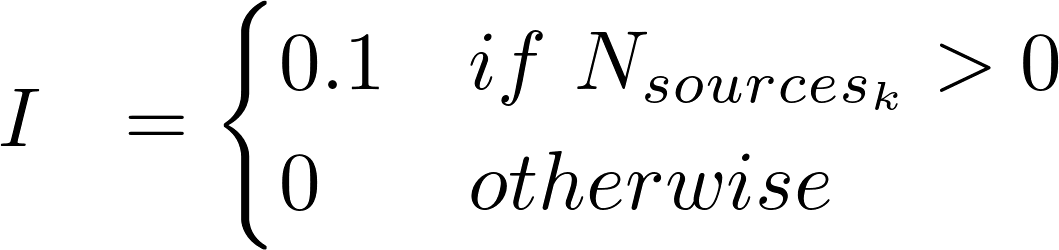
where:
k ∈ HPO, CLINVAR, GWASCAT, GWASDB

where:
Npubs is the number of publications supporting a GDA in the sources LHGDN and BEFREE
Distribution of the DisGeNET score for GDAs according to the number of sources reporting the association
VDA Score
The DisGeNET Score (S) for VDAs is computed according to:
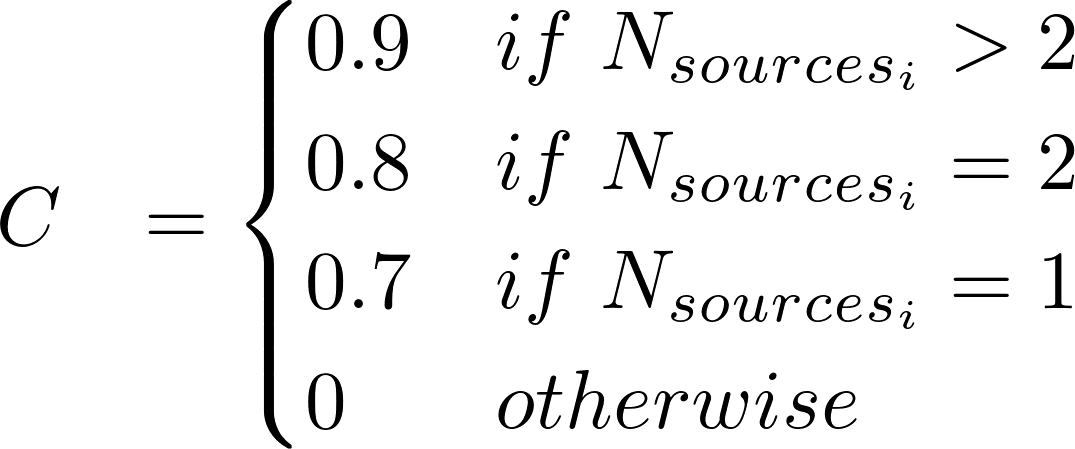
where:
Nsourcesi is the number of CURATED sources supporting a VDA
i ∈ UNIPROT,CLINVAR, GWASCAT, GWASDB

where:
Npubs is the number of publication supporting a VDA in the source in BeFree
Distribution of the DisGeNET Score for VDAs according to the number of sources reporting the association
Disease Specificity Index
There are genes (or variants) that are associated wiht multiple diseases (e.g. TNF) while others are associated with a small set of diseases or even to a single disease. The Disease Specificity Index (DSI) is a measure of this property of the genes (and variants). It reflects if a gene (or variant) is associated to several or fewer diseases. It is computed according to:
-
- N
d is the number of diseases associated to the
gene/variant
- N T is the total number of diseases in DisGeNET
The DSI ranges from 0.25 to 1. Example: TNF, associated to more than 1,500 diseases, has a DSI of 0.263, while HCN2 is associated to one disease, with a DSI of 1.
If the DSI is empty, it implies that the gene/variant is associated only to phenotypes.
Disease Pleiotropy Index
The rationale is similar than for the DSI, but we consider if the multiple diseases associated to the gene (or variant) are similar among them (belong to the same MeSH disease class, e.g. Cardiovascular Diseases) or are completely different diseases and belong to different disease classes. The Disease Pleiotropy Index (DPI) is computed according to:
-
- N
dc is the number of the different MeSH disease classes
of the diseases associated to the gene/variant
- N TC is the total number of MeSH diseases classes in DisGeNET (29)
The DPI ranges from 0 to 1. Example: gene KCNT1 is associated to 39 diseases, 4 disease groups, and 18 phenotypes. 29 out of the 39 diseases have a MeSH disease class. The 29 diseases are associated to 5 different MeSH classes. The DPI index for KCNT1 = 5/29 ~ 0.172. Nevertheless, gene APOE, associated to more than 700 diseases of 27 disease classes has a DPI of 0.931.
If the gene/variant has no DPI value, it implies that the gene/variant is associated only to phenotypes, or that the associated diseases do not map to any MeSH classes.
Evidence Level
The Evidence Level (EL) is a metric developed by ClinGen that measures the strength of evidence of a gene-disease relationship that correlates to a qualitative classification: "Definitive", "Strong", "Moderate", "Limited", "Disputed" (Strande et al., 2017). GDAs that have been reported by ClinGen will have their corresponding Evidence Level. Furthermore, we have adapted a similar metric reported by Genomics England PanelApp to correspond to the same categories from ClinGen: GDAs marked by Genomics England PanelApp as High Evidence are labeled as strong in DisGeNET. Those labeled as Moderate Evidence are labeled as moderate and LowEvidence associations are labeled as limited.
Evidence Index
The "Evidence index" (EI) indicates the existence of contradictory results in publications supporting the gene/variant-disease associations. This index is computed for the sources BeFree and PsyGeNET, by identifying the publications reporting a negative finding on a particular VDA or GDA. Note that only in the case of PsyGeNET, the information used to compute the EI has been validated by experts. The EI is computed as follows:
where:
Npubspositive is the number of publication supporting a GDA in BeFree or PsyGeNET, or a VDA in BeFree
Npubstotal is the total number of publications in BeFree or PsyGeNET supporting that GDA, or in BeFree for VDAs
Data Attributes
In order to ease the interpretation and analysis of gene-disease, variant-disease associations, and disease-disease associations we provide the following information for the data.
Genes
For human genes, HGNC symbols, and Uniprot accession numbers (used by Uniprot) are converted to NCBI Entrez gene identifiers using an in house dictionary that cross references HGNC, Uniprot and NCBI-Gene information. For mapping of mouse and rat genes, we used files HOM_MouseHumanSequence, and RGD_ORTHOLOGS, both with information of orthology from MGD and RGD, respectively to map rat and mouse Entrez gene identifiers to human Entrez identifiers. We discarded the associations involving rat or mouse genes without a human ortholog.
Genes in DisGeNET are annotated with:
- the official gene symbol, from the NCBI
- the NCBI Official Full Name
- the Uniprot accession
- the Disease Specificity Index (DSI)
- the Disease Pleiotropy Index (DPI)
- the pLI, defined as the probability of being loss-of-function intolerant, is a gene constraint metric provided by the GNOMAD consortium. A gene constraint metric aims at measuring how the naturally occurring LoF (loss of function) variation has been depleted from a gene by natural selection (in other words, how intolerant is a gene to LoF variation). LoF intolerant genes will have a high pLI value (>=0.9), while LoF tolerant genes will have low pLI values (<=0.1). The LoF variants considered are nonsense and essential splice site variants.
- the top level category from the Drug Target Ontology.
Variants
Variants in DisGeNET are annotated with:- The position in the chromosome
- The reference and alternative alleles
- The class of the variant: SNP, deletion, insertion, indel, somatic SNV, substitution, sequence alteration, and tandem repeat These attributes are retrieved from dbSNP, the NCBI Short Genetic Variations database a catalog of short variations in nucleotide sequences from a wide range of organisms ( Sherry, et al., 2001 ). The data was retrieved in January 2020 (corresponding to NCBI dbSNP Human Build 153, and to Assembly GRCh38).
- The allelic frequency in genomes and exomes according to GNOMAD. The Genome Aggregation Database is a resource that aggregates and harmonizes both exome and genome sequencing data from a wide variety of large-scale sequencing projects ( Exome Aggregation Consortium, 2016). The data spans 125,748 exomes and 71,702 genomes from unrelated individuals sequenced as part of various disease-specific and population genetic studies ( release 2.1.1 for exomes and 3.0 for genomes).
- The most severe consequence type according to the Variant Effect Predictor The Ensembl Variant Effect Predictor determines the effect of a variant, or a list of variants (SNPs, insertions, deletions, CNVs or structural variants) on genes, transcripts, and protein sequence, as well as regulatory regions ( McLaren et al, 2016 ). We use the Ensembl API (release 11.2) to obtain the most severe consequence type of the variant.
- the gene correspoding to the consequence type assigend by VEP
- the Disease Specificity Index (DSI)
- the Disease Pleiotropy Index (DPI)
Diseases
The vocabulary used for diseases in DisGeNET are the Concept Unique Identifiers (CUIs) from the Unified Medical Language System®(UMLS) Metathesaurus®(version UMLS 2019AA). The repositories of gene-disease associations use different disease vocabularies, OMIM® terms for diseases from UniProt, CTDTM, and MGD; MeSH terms used by CTDTM, LHGDN, and RGD; MONDO for ClinGen; HPO identifiers for HPO, UMLS® CUIs from CLINVAR and PsyGeNET; EFO for the GWAS Catalog; Orphanet identifiers are mapped using Orphanet cross-references and MESH, EFO and DO vocabularies for GWASdb. Disease names from the Genomics England PanelApp, and the Cancer Genome Interpreter are normalized using the UMLS Metathesaurus. We also used UMLS® Metathesaurus® concept structure to map OMIM, HPO and MeSH terms to UMLS® CUIs.
Diseases in DisGeNET are annotated with:
- the disease name, provided by the UMLS® Metathesaurus®
- the UMLS® semantic types
- the MeSH class: We classify the diseases according the MeSH hierarchy using the upper level concepts of the MeSH tree branch C (Diseases) plus three concepts of the F branch (Psychiatry and Psychology: "Behavior and Behavior Mechanisms", "Psychological Phenomena and Processes", and "Mental Disorders").
- The top level concepts from the Human DiseaseOntology.
- The DisGeNET disease type: disease, phenotype and group.
We consider a disease entries mapping to the following UMLS® semantic types:
-
- Disease or Syndrome
- Neoplastic Process
- Acquired Abnormality
- Anatomical Abnormality
- Congenital Abnormality
- Mental or Behavioral Dysfunction
We consider a phenotype entries mapping to the following UMLS® semantic types:
-
- Pathologic Function
- Sign or Symptom
- Finding
- Laboratory or Test Result
- Individual Behavior
- Clinical Attribute
- Organism Attribute
- Organism Function
- Organ or Tissue Function
- Cell or Molecular Dysfunction
These classifications were manually checked. In addition, disease entries referring to disease groups such as "Cardiovascular Diseases", "Autoimmune Diseases", "Neurodegenerative Diseases, and "Lung Neoplasms" were classified as disease group .
Additionally, we have removed terms considered as diseases by other sources, but are not strictly diseases, such as terms belonging to the following UMLS® semantic types:
-
- Gene or Genome
- Genetic Function
- Immunologic Factor
- Injury or Poisoning
Gene-Disease Associations
- the DisGeNET score
- the DisGeNET Gene-Disease Association Type
- the Evidence Level
- the Evidence Index
- the year initial: First time that the association was reported
- the year final: Last time that the association was reported
- the publication(s) that reports the gene-disease association, with the Pubmed Identifier
- a representative sentence from the publication describing the association between the gene and the disease (If a representative sentence is not found, we provide the title of the paper)
- the original source reporting the Gene-Disease Association
For a seamless integration of gene-disease association data, we developed the DisGeNET association type ontology. All association types as found in the original source databases are formally structured from a parent GeneDiseaseAssociation class if there is a relationship between the gene/protein and the disease, and represented as ontological classes. For more information, see here.
Variant-Disease Associations
- the DisGeNET score
- the Evidence Index
- the publication(s) that reports the variant-disease association, with the Pubmed Identifier
- the year initial: First time that the association was reported
- the year final: Last time that the association was reported
- a representative sentence from the publication describing the association between the variant and the disease (If a representative sentence is not found, we provide the title of the paper)
- the original source reporting the Variant-Disease Association
Disease-Disease Associations
- Jaccard Index based on shared genes
- p-value based on shared genes
- Jaccard Index based on shared variants
The DisGeNET Association Type Ontology
For a seamless integration of gene-disease association data, we developed the DisGeNET association type ontology. All association types as found in the original source databases are formally structured from a parent GeneDiseaseAssociation class if there is a relationship between the gene/protein and the disease, and represented as ontological classes. It is an OWL ontology that has been integrated into the Sematicscience Integrated Ontology (SIO), which is an OWL ontology that provides essential types and relations for the rich description of objects, processes and their attributes [PDF]. You can check SIO gene-disease association classes from this URL or download the entire SIO OWL-DL ontology file . The SIO ontology can be also accessed at the NCBO Bioportal. DisGeNET GDAs in RDF are semantically harmonized using SIO classes.
The DisGeNET association type ontology is depicted below.
The description of each association type in our ontology is:
- Therapeutic: This relationship indicates that the gene/protein has a therapeutic role in the amelioration of the disease.
- Biomarker: This relationship indicates that the gene/protein either plays a role in the etiology of the disease (e.g. participates in the molecular mechanism that leads to disease) or is a biomarker for a disease.
- Genomic Alterations: This relationship indicates that a genomic alteration is linked to the gene associated with the disease phenotype.
- GeneticVariation: This relationship indicates that a sequence variation (a mutation, a SNP) is associated with the disease phenotype, but there is still no evidence to say that the variation causes the disease.
- Causal Mutation: This relationship indicates that there are allelic variants or mutations known to cause the disease.
- Germline Causal Mutation: This relationship indicates that there are germline allelic variants or mutations known to cause the disease, and they may be passed on to offspring.
- Somatic Causal Mutation: This relationship indicates that there are somatic allelic variants or mutations known to cause the disease, but they may not be passed on to offspring.
- Chromosomal Rearrangement: This relationship indicates that a gene is included in a chromosomal rearrangement associated with a particular manifestation of the disease.
- Fusion Gene: This relationship indicates that the fusion between two different genes (between promoter and/or other coding DNA regions) is associated with the disease.
- Susceptibility Mutation: This relationship indicates that a gene mutation in a germ cell that predisposes to the development of a disorder, and that is necessary but not sufficient for the manifestation of the disease.
- Modifying Mutation: This relationship indicates that a gene mutation is known to modify the clinical presentation of the disease.
- Germline Modifying Mutation: This relationship indicates that a germline gene mutation modifies the clinical presentation of the disease, and it may be passed on to offspring.
- Somatic Modifying Mutation: This relationship indicates that a somatic gene mutation modifies the clinical presentation of the disease, but it may not be passed on to offspring.
- AlteredExpression: This relationship indicates that an altered expression of the gene is associated with the disease phenotype.
- Post-translational Modification: This relationship indicates that alterations in the function of the protein by means of post-translational modifications (methylation or phosphorylation of the protein) are associated with the disease phenotype.
The labels from the original sources are mapped to DisGeNET Gene-Disease Ontology according to:
Type of data | Association Type | Original Source Label |
GDAS | Altered Expression | BeFree (Altered Expression) |
LHGDN (Altered Expression) | ||
Biomarker | BeFree (BioMarker) | |
ClinGen | ||
CTD (marker/mechanism) | ||
Genomics England | ||
HPO | ||
LHGDN (BioMarker) | ||
MGD | ||
RGD | ||
PsyGeNET | ||
CausalMutation | CGI (mutation) | |
ClinVar (Pathogenic) | ||
Chromosomal Rearrangement | ORPHANET (Role in the phenotype of) | |
Fusion Gene | ORPHANET (Part of a fusion gene in) | |
Genetic Variation | BeFree (Genetic Variation) | |
ClinVar (Affects, association, Likely pathogenic, Not provided, other) | ||
LHGDN (Genetic Variation) | ||
GWAS Catalog | ||
GWASdb | ||
ORPHANET (Candidate gene tested in) | ||
UniProt | ||
GenomicAlterations | CGI (amplification, deletion) | |
Germline Causal Mutation | ORPHANET (Disease-causing germline mutation(s) in, Disease-causing germline mutation(s) (gain of function) in, Disease-causing germline mutation(s) (loss of function) in) | |
Germline Modifying Mutation | ORPHANET (Modifying germline mutation in) | |
Modifying Mutation | RGD (severity, disease_progression, onset) | |
PostTranslational Modification | BeFree (PostTranslational Modification) | |
LHGDN (PostTranslational Modification) | ||
Somatic Causal Mutation | ORPHANET (Disease-causing somatic mutation(s) in) | |
Somatic Modifying Mutation | ORPHANET (Modifying somatic mutation in) | |
Susceptibility Mutation | CLINVAR (confers sensitivity, risk factor) | |
ORPHANET (Major susceptibility factor in) | ||
Susceptibility (RGD) | ||
Therapeutic | CTD (therapeutic) | |
RGD (treatment) | ||
VDAs | CausalMutation | ClinVar (Pathogenic) |
SusceptibilityMutation | CLINVAR (confers sensitivity, risk factor) | |
Genetic Variation | BeFree (Genetic Variation) | |
ClinVar (Affects, association, Likely pathogenic, Not provided, other) | ||
GWAS Catalog | ||
GWASdb | ||
UniProt |
Version History
May 4, 2020- DisGeNET Database 7.0 released
- All data sources were updated
- Risk allele of the disease variant now available for ClinVar, the GWAS Catalog and GWASdb
- Added the expansion of the disease search using semantic similarity
- Updated platform (v1.1.0)
- Added Disease-Disease Associations (DDAs)
- Added DisGeNET REST API (v1.0.0)
- Updated disgenet2r package
February 12, 2019
- New attributes added to bulk file downloads.
January 14, 2019
- Launched new platform (v1.0.0)
- DisGeNET Database 6.0 released
- All data sources were updated
- New data sources added: CGI, ClinGen, Genomics England PanelApp, GWAS db
- Evidence Level to classify GDAs was added
- New aggregated data source: INFERRED, containing data from HPO, GWAS catalog, and GWASdb
- New data for genes: the probability of being loss-of-function intolerant according to GNOMAD data
- New data for variants: the allelic frequency according to GNOMAD data
- Improvements to BeFree
DisGeNET 5.0 - May 28, 2017
- All data sources were updated
- New data sources added: PsyGeNET, HPO
- New score to rank VDAs
- New: Specificity and Pleiotropy indexes for variants were added
DisGeNET 4.0 - October, 2016
- 254 clinical concepts were reclassified as "group"
DisGeNET 4.0 - June, 2016
- New entry point in the web interface for variants
- New data for variants: the chromosomal coordinates, and the reference and alternative alleles
- New data for variants: the class of the variant: SNP, deletion, insertion, indel, somatic SNV, substitution, sequence alteration, and tandem repeat
- New data for variants: the allelic frequency according to the 1000 Genomes Project and Exome Aggregation Consortium
- New data for variants: the most severe consequence type according to the VEP
DisGeNET 4.0 - April 15, 2016
- All data sources were updated
- New data sources added: Orphanet, GWAS Catalog
- New association types added to the DisGeNET GDA ontology
- New disease annotations added to the browser: HPO,and HDO
- New disease classification: disease, phenotype, and group
- New: Specificity and Pleiotropy indexes for genes were added
- Information on SNP-gene and SNP-disease association is now available
DisGeNET 3.0 - May 15, 2015
- All data sources were updated
- New data source added: ClinVar
- Improved text mining data: GDAs from BeFree classified by association type
- More information on SNPs: links to dbSNP, ENSEMBL, and ClinVar
DisGeNET 2.1 - May 5, 2014
- Second release of DisGeNET as Linked Data (DisGeNET RDF v2.1.0)
- New text mining information using the BeFree System
DisGeNET 2.0 - February 5, 2014
- First release of DisGeNET as Linked Data (DisGeNET RDF v1.0)
- Added information about rat disease models from CTDTM and RGD
- New Text mining information
DisGeNET - July 20, 2012
- Added information about mouse disease models from CTDTM and MGD
- Changed disease identifiers from MeSH, OMIM® to UMLS® CUIs
- DisGeNET web interface is launched
DisGeNET 1.02 - Oct 7th 2010
- added README.txt
- changed citation of application note
- fixed a bug in build script which did not copy the images
- added plugin.props so DisGeNET properly shows up in the Cytoscape plugin manager
DisGeNET 1.01 - Oct 4th 2010
- fixed minor bug, two columns (pmids, sentence) were mixed up in the database table geneDiseaseNetwork
- build script added to source code
DisGeNET 1.0 - Sep 21st 2010
- initial release of DisGeNET as a Cytoscape plugin and SQLite database